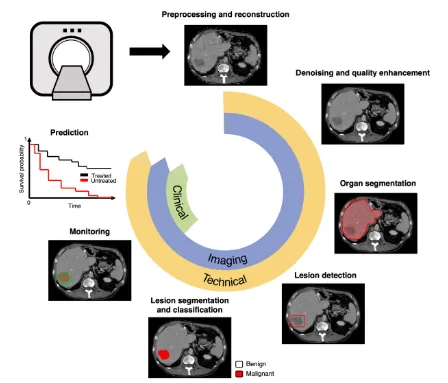
In the past decade, deep learning architectures, which essentially consist of neural networks with numerous layers, have emerged as a dominant class of machine learning algorithms. Owing to the availability of larger datasets in radiology and access to high-performance graphical processing units, deep learning has provided state-of-the-art performance for various computer vision tasks such as lesion detection, segmentation, classification, monitoring, and prediction of treatment response.
Because of the open access to deep neural network architectures and the numerous clinical use scenarios already reported in the radiology literature, we anticipate that deep learning will be highly prevalent in machine learning publications in the near future. However, to any radiologist who would like to start a deep learning project ‘from scratch’ by exploiting institutional data, the path may be unclear and rugged.
In this paper, we provide a roadmap for radiologists or teams eager to undertake a deep learning-based project. For educational purposes, we describe a prototypical workflow for liver lesion detection, segmentation, classification, monitoring, and prediction of tumor recurrence and patient survival. For convenience, we included a structured checklist. We also discussed challenges, including ethical considerations, cohorting, data collection, anonymization, and availability of expert annotations. This practical guidance may be adapted to any project that requires automated medical image analysis.
Key points
· Deep learning provides state-of-the-art performance for detection, segmentation, classification, and prediction.
· A multi-disciplinary team with clinical, imaging, and technical expertise is recommended.
· Data collection and curation constitute the most time-consuming steps.
· Several open-source deep learning frameworks with permissive licenses are available.
· Cloud computing leverages third-party hardware, storage, and technical resources.